ESG Data Analysis: How AI Evaluates Sustainable Investments and Companies
This blog post explores the transformative role of Artificial Intelligence (AI) in ESG (Environmental, Social, Governance) data analysis. It highlights how AI enhances the evaluation of sustainable investments by automating data collection, processing, and interpretation, thus addressing challenges like data quality, subjectivity, and scalability. The post discusses key AI technologies such as Natural Language Processing (NLP), machine learning, and predictive analytics, which improve the accuracy and efficiency of ESG assessments. It also emphasizes the importance of AI in overcoming the limitations of traditional methods and facilitating better decision-making in sustainable investments.
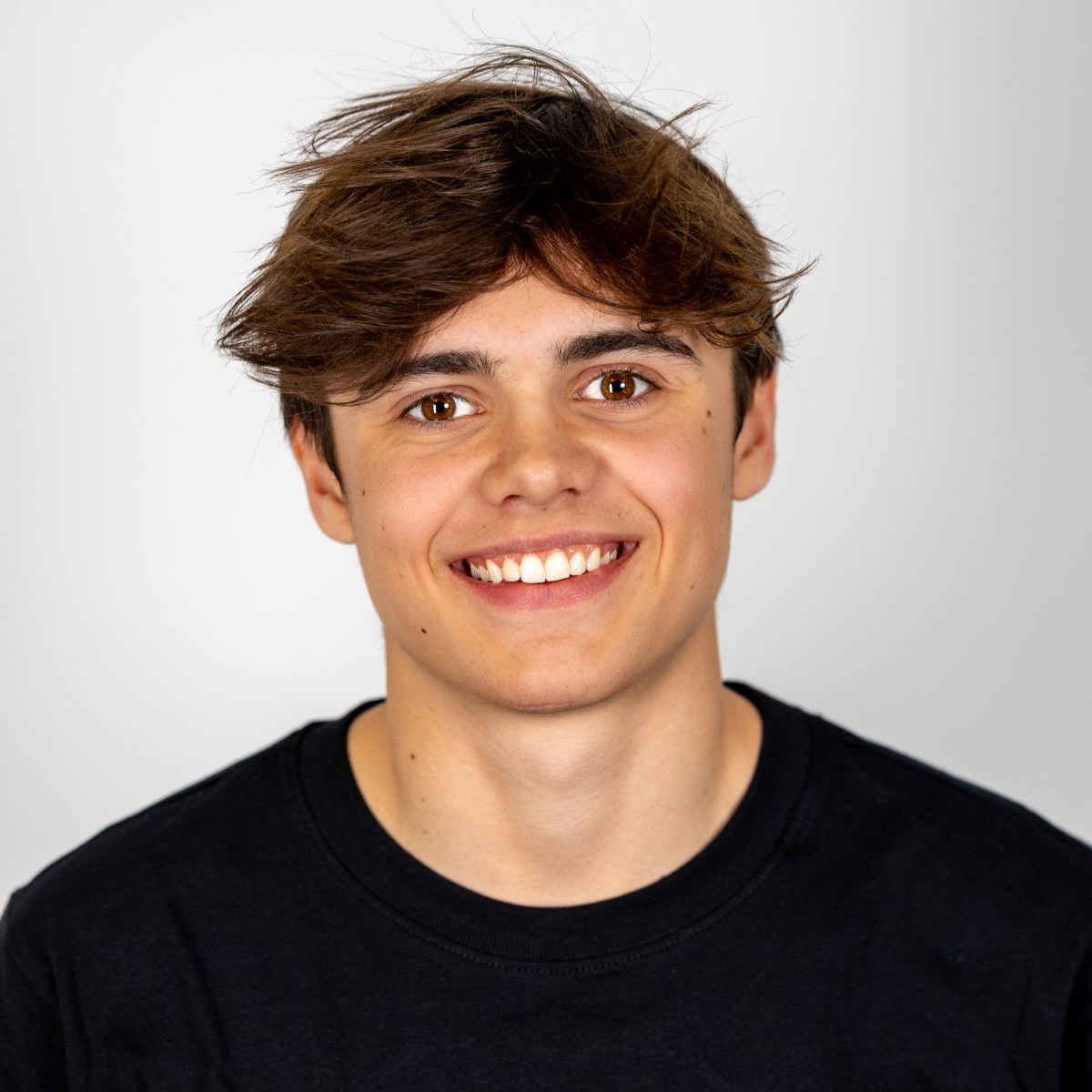
Introduction: The Revolution of Sustainability Assessment through Artificial Intelligence
In an era where sustainability is increasingly becoming a critical factor for corporate success and investment decisions, Artificial Intelligence is revolutionizing the way we analyze and interpret ESG (Environmental, Social, Governance) data. This technological evolution allows investors, companies, and stakeholders to make informed decisions that are not only based on financial metrics but also consider environmental, social, and governance aspects. The combination of big data, machine learning, and AI-driven analytical tools opens up new dimensions in sustainability assessment, offering a more precise and objective view of companies' actual performance in relation to sustainability.
This article explores how modern AI technologies are transforming ESG data analysis, the methods used, and how they can improve decision-making for sustainable investments. We will examine the fundamentals of ESG evaluation, the role of data analysis, and the growing importance of AI in identifying and assessing sustainable companies and investment opportunities.
The Basics: What is ESG and Why is It So Important Today?
ESG stands for Environmental, Social, and Governance and provides a comprehensive framework for evaluating a company's sustainability performance. These three pillars have become the standard criteria for sustainable investments and corporate assessments. But what exactly do we understand by these three components?
- Environmental (E): This aspect includes factors like greenhouse gas emissions, resource consumption, waste management, and a company's environmental policies.
- Social (S): This aspect evaluates relationships with employees, customers, suppliers, and communities, covering topics such as labor standards, diversity, and inclusion.
- Governance (G): This focuses on corporate governance, including board structure, compensation practices, transparency, and ethical business practices.
The importance of ESG has grown exponentially in recent years. According to PwC, more than 75% of institutional investors already integrate ESG criteria into their investment decisions. This trend is driven by several factors: First, there is increasing awareness of the impact corporate practices have on the environment and society. Second, studies show that ESG-focused companies often perform better in the long term and are more resilient to market volatility.
"ESG factors are no longer just a fringe issue, but increasingly a central component of risk assessment and investment decisions," confirms an analysis by EcoVadis. The integration of ESG criteria serves not only to minimize risks but also to identify opportunities and competitive advantages in a changing economic landscape.
Also noteworthy is the increasing regulatory relevance: The EU Taxonomy, the Disclosure Regulation (SFDR), and other frameworks like the Corporate Sustainability Reporting Directive (CSRD) are creating a binding framework for ESG reporting. These regulatory requirements accelerate the need for precise, comparable, and transparent ESG data—a challenge in which AI increasingly plays a key role.
ESG Data Analysis: Fundamentals and Challenges
ESG data analysis forms the foundation for evaluating the sustainability performance of companies. It involves the systematic collection, processing, and interpretation of data on environmental, social, and governance aspects. These data come from various sources and are available in different formats, making analysis complex.
The Diversity of ESG Data Sources
ESG data is gathered from a variety of sources, providing both structured and unstructured information. Key sources include:
- Corporate reports and disclosures (sustainability reports, annual reports, integrated reports)
- Regulatory submissions and compliance documentation
- Company-specific environmental and social data (e.g., CO2 emissions, water consumption, workplace accidents)
- External assessments and ratings by specialized ESG data providers
- Media reports and news
- Social media and public discussions
- Satellite imagery and IoT data for environmental monitoring
Integrating these heterogeneous data sources presents a significant challenge that traditional analysis methods often struggle to handle. This is where moderne ESG-Data-Governance comes in, offering a structured framework for managing, quality-assuring, and analyzing ESG data.

Key Challenges of ESG Data Analysis
ESG data analysis is associated with specific challenges that make the use of advanced technologies like AI essential:
- Data Quality and Availability: ESG data is often incomplete, inconsistent, or difficult to access. Particularly in smaller companies or certain regions, there are significant data gaps.
- Standardization Issues: Despite efforts for standards like GRI (Global Reporting Initiative) or SASB (Sustainability Accounting Standards Board), there is a lack of unified reporting formats and metrics.
- Subjectivity and Bias: Traditional ESG assessments are often vulnerable to subjective interpretations and methodological biases.
- Dynamic Nature: ESG factors and their importance continuously evolve, causing static analysis models to quickly become outdated.
- Scalability: Manual analysis of the growing amount of data is time-consuming and costly.
These challenges highlight the need for innovative solutions capable of efficiently processing and analyzing large data volumes. This is where AI comes into play, leveraging machine learning, natural language processing, and other advanced techniques to unlock new possibilities in ESG data analysis.
"The implementation of effective ESG data governance is key to overcoming these challenges," emphasizes a PwC study. It not only ensures compliance with regulatory requirements but also lays the foundation for data-driven decision-making and strategic planning in sustainability.
AI as a Game-Changer: How Artificial Intelligence is Revolutionizing ESG Analysis
Artificial Intelligence is fundamentally transforming ESG data analysis, offering new dimensions of precision, efficiency, and insight. By combining various AI technologies, challenges that were difficult to overcome with traditional analysis methods can now be addressed.
Key Technologies and Their Application in ESG Analysis
- Natural Language Processing (NLP): This technology enables the analysis of unstructured text data such as sustainability reports, media articles, and social media posts to extract relevant ESG information. NLP algorithms can capture tone, context, and relevance of texts, helping to identify controversies or positive ESG developments.
- Machine Learning (ML): ML algorithms can recognize patterns in large datasets and develop predictive models that identify ESG risks and opportunities early. By continuously learning from new data, these models refine their predictions over time.
- Computer Vision: This technology analyzes visual data, such as satellite imagery, to monitor and quantify environmental impacts (e.g., deforestation, water pollution), even in regions with limited direct reporting.
- Deep Learning: Complex neural networks can model non-linear relationships between ESG factors and company performance, providing deeper insights into the material impacts of sustainability aspects.
- Predictive Analytics: Advanced forecasting methods can anticipate future ESG trends and risks, enabling a proactive rather than reactive approach to sustainability management.

Practical Applications of AI in ESG Analysis
The practical application of AI in ESG analysis spans several areas:
- Automated ESG Scoring Systems: AI systems can analyze thousands of data points from various sources to generate comprehensive ESG scores, taking industry-specific factors into account and enabling comparisons of companies within their peer group.
- Real-Time Controversy Detection: AI-driven tools continuously monitor news, social media, and other sources to identify ESG-related controversies that traditional analyses might overlook. These early warning systems allow investors and companies to react quickly to potential risks.
- Supply Chain Analysis: AI algorithms can analyze complex supply chains to uncover sustainability risks, such as human rights violations or environmental damage. This technology enhances transparency and accountability across the entire value chain.
- Sentiment Analysis: NLP-based sentiment analysis evaluates the public perception of companies regarding ESG issues, providing valuable insights into reputational risks and opportunities.
"AI systems enable a depth and breadth of ESG analysis that conventional methods cannot achieve," emphasizes an analysis by Sustainable. "They can capture not only obvious sustainability indicators but also subtle correlations and trends that can be crucial for long-term performance."
Integrating AI into ESG analysis offers numerous benefits, including greater objectivity, improved scalability, enhanced data quality through automated validation, and the ability to make forward-looking rather than retrospective assessments. These advantages make AI an indispensable tool for modern ESG analysts and investors.
Our Sustainability Indicator: AI-Driven Analysis of Corporate Communication
Our webAI Sustainability Indicator offers an innovative method for evaluating the sustainability communication of companies. The technology has been validated in collaboration with the OECD and independent experts and allows for an objective assessment of sustainability intensity based on publicly available corporate websites.
Methodology: How the Indicator Evaluates Sustainability
Our approach involves a multi-stage analysis:
- Keyword-Based Recognition: webAI scans corporate websites for specific sustainability terms related to key ESG topics such as circular economy, energy transition, renewable energy, resource efficiency, emission reduction, and recycling.
- Context and Relevance Check: AI not only assesses the presence of these terms but also analyzes the surrounding paragraphs to ensure that sustainability is a substantive focus and not just superficially mentioned.
- Sustainability Score Calculation: Based on the proportion of sustainability-related content relative to the total text on the corporate website, a Sustainability Score is calculated.
- Categorization of Sustainability Intensity: Companies are classified into different intensity levels based on their score, enabling a differentiated view of their sustainability communication.
Our Sustainability Intensity Scores were also validated in a study on “Greenwashing in the U.S. Metals Industry” published by researchers from the University of Salzburg, University of Heidelberg, Harvard University and the University of Giessen in the prestigious journal Science of The Total Environment.
Data Acquisition and Quality: The Foundation for Reliable ESG Ratings
The quality of ESG analysis is heavily dependent on the availability and reliability of underlying data. The financial industry faces particular challenges in the area of ESG data, challenges that AI technologies are increasingly addressing.
The Role of ESG Data Providers
ESG data providers play a central role in the sustainability assessment ecosystem. They collect, structure, and analyze data from various sources to provide standardized ESG ratings and metrics. Leading providers include MSCI ESG Research, Sustainalytics, ISS ESG, Bloomberg ESG, and Refinitiv. These providers offer investors and companies structured ESG ratings that serve as the foundation for sustainable investment decisions. However, challenges remain: Data aggregation and evaluation methods often differ, leading to inconsistencies in ESG ratings. Moreover, gaps still exist in the coverage of certain regions or sectors, making comprehensive and precise ESG assessments more difficult.
How AI Improves ESG Data Quality
Artificial Intelligence offers innovative solutions for improving ESG data quality. Through automated data validation, AI algorithms can detect inconsistencies in reports, correct erroneous data, and fill in missing values through intelligent estimations. Additionally, machine learning enables the harmonization of different data sources by identifying patterns and correlations that allow for a more consistent evaluation.
Another advantage is AI’s ability to integrate real-time data into ESG assessments. While traditional ESG analyses often rely on historical reports, AI-driven systems can incorporate current developments from social media, news, or environmental changes directly into the evaluation, ensuring a more dynamic and accurate assessment of a company's sustainability performance.
The Future of ESG Analysis: Opportunities and Challenges
With the increasing integration of AI in ESG analysis, numerous opportunities arise. Investors can make more informed decisions, companies gain deeper insights into their sustainability performance, and regulatory authorities benefit from better data transparency. However, challenges remain, particularly in addressing ethical concerns in AI deployment, the need for standardized ESG data structures, and ensuring data security and privacy.
Conclusion: ESG Analysis in the Age of AI
The integration of Artificial Intelligence into ESG data analysis marks a milestone in evaluating sustainable companies and investments. By automating and refining data evaluation, AI helps enhance the objectivity and efficiency of ESG ratings. Nevertheless, establishing standards and ensuring the transparency of evaluation methods remain crucial. The future of sustainable investments will largely depend on how well technology is integrated with responsible ESG strategies.
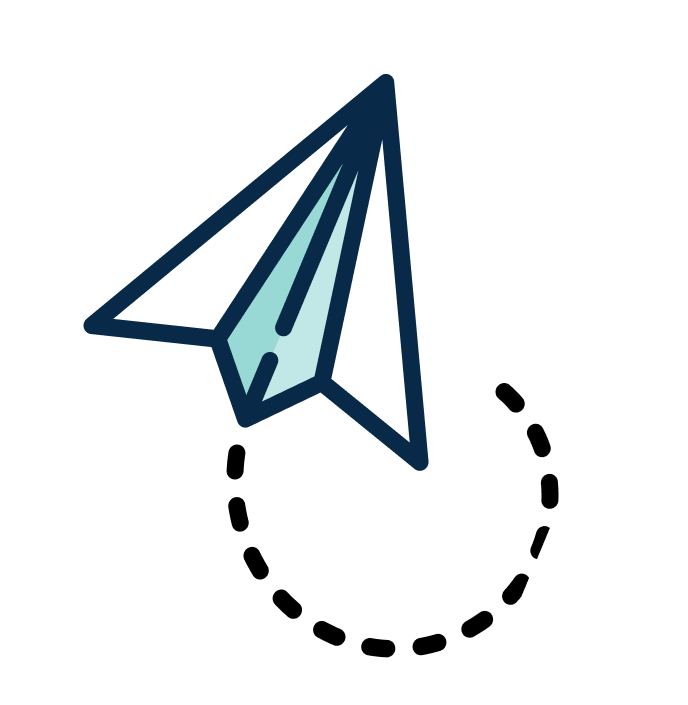