Ensuring Data Quality in AI-Powered Market Analysis
High-quality data forms the foundation for successful AI-driven market analysis. Companies that invest in data quality and a clear data strategy lay the groundwork for more precise insights and sustainable competitive advantages.
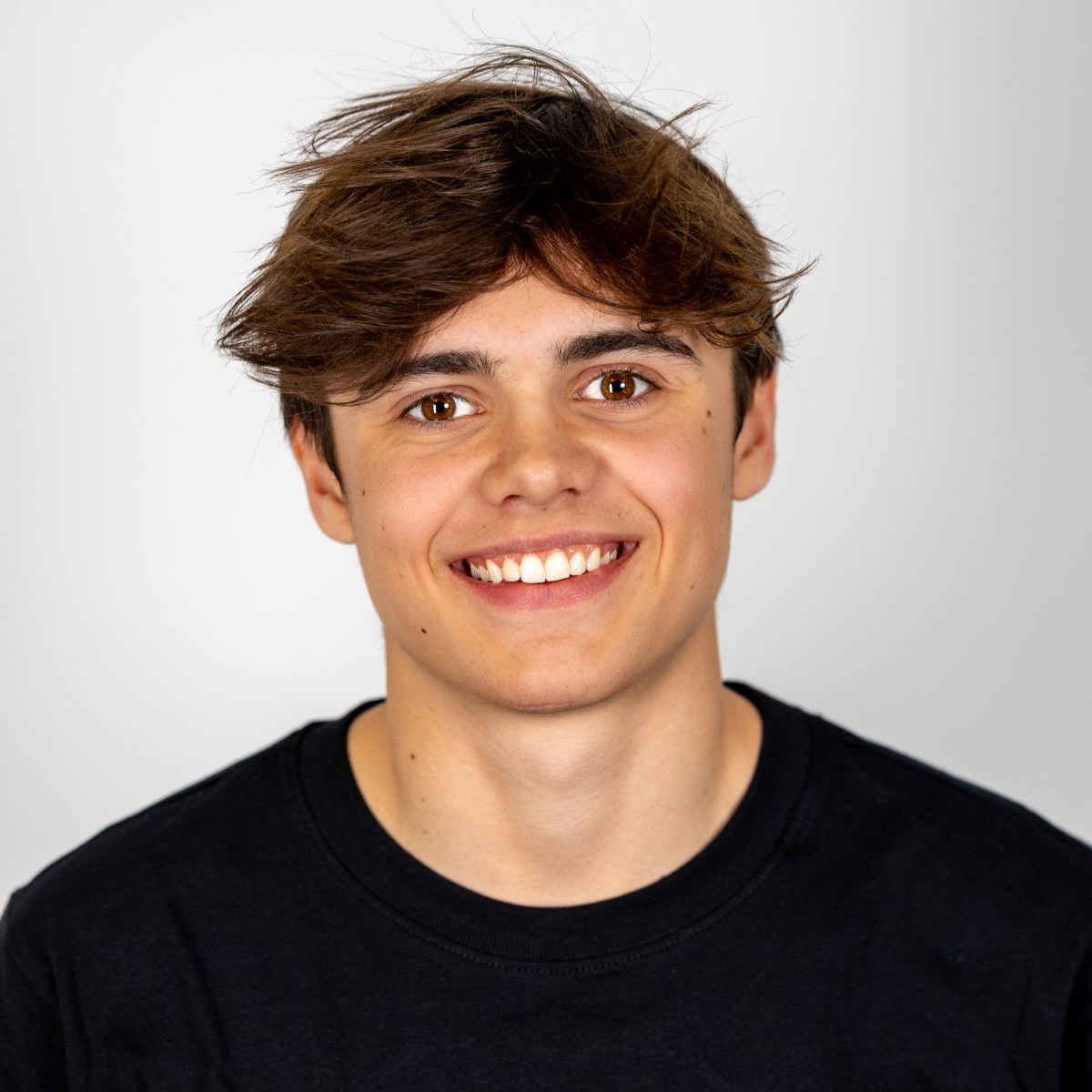
In an era where Artificial Intelligence is increasingly revolutionizing how companies conduct market analyses, one factor is often underestimated: the quality of the underlying data. While algorithms and AI models become more sophisticated, the old adage "Garbage In, Garbage Out" remains unchanged in its relevance. The most advanced AI systems cannot deliver reliable insights if they must work with inferior data.
Market analysis has fundamentally changed. It's no longer just the quantity of available data, but its quality that determines the success of strategic decisions. In Germany, where precision is traditionally valued, this topic is gaining increasing importance for companies of all sizes.
What is Data Quality?
Data quality refers to the suitability of data for its intended purpose in the context of business operations, decision-making, and planning. It's not just about the absence of errors, but rather about the ability of data to provide reliable and valuable insights. A synonym for data quality could be "data information value" – a term that emphasizes the importance of the actual usefulness and applicability of data.
Outcome quality, a closely related term, refers specifically to the reliability and validity of insights gained through data analyses. It is the direct result of the underlying data quality and the analytical methods applied.
Understanding the Dimensions of Data Quality
Data quality encompasses various dimensions that together provide a comprehensive picture of the data's suitability for analytical purposes. To effectively manage data quality, companies must know these dimensions and monitor them continuously.
What are the 5 Elements of Data Quality?
Five fundamental elements are particularly crucial for assessing data quality:
Accuracy refers to the correctness and precision of the data. In market analysis, this means, for example, that customer data, sales figures, or market shares are recorded without errors and exactly.
Completeness concerns the question of whether all necessary data points are present. Gaps in datasets can lead to distorted analyses, as AI models may miss or misinterpret important information.
Consistency describes the freedom from contradictions in the data across different systems and points in time. Inconsistencies often arise when data from different sources are merged.
Timeliness refers to the temporal proximity of the data. Especially in dynamic markets, outdated data can lead to false conclusions.
Relevance considers the appropriateness of the data for the specific analysis question. Not all available data is equally relevant for every market analysis.
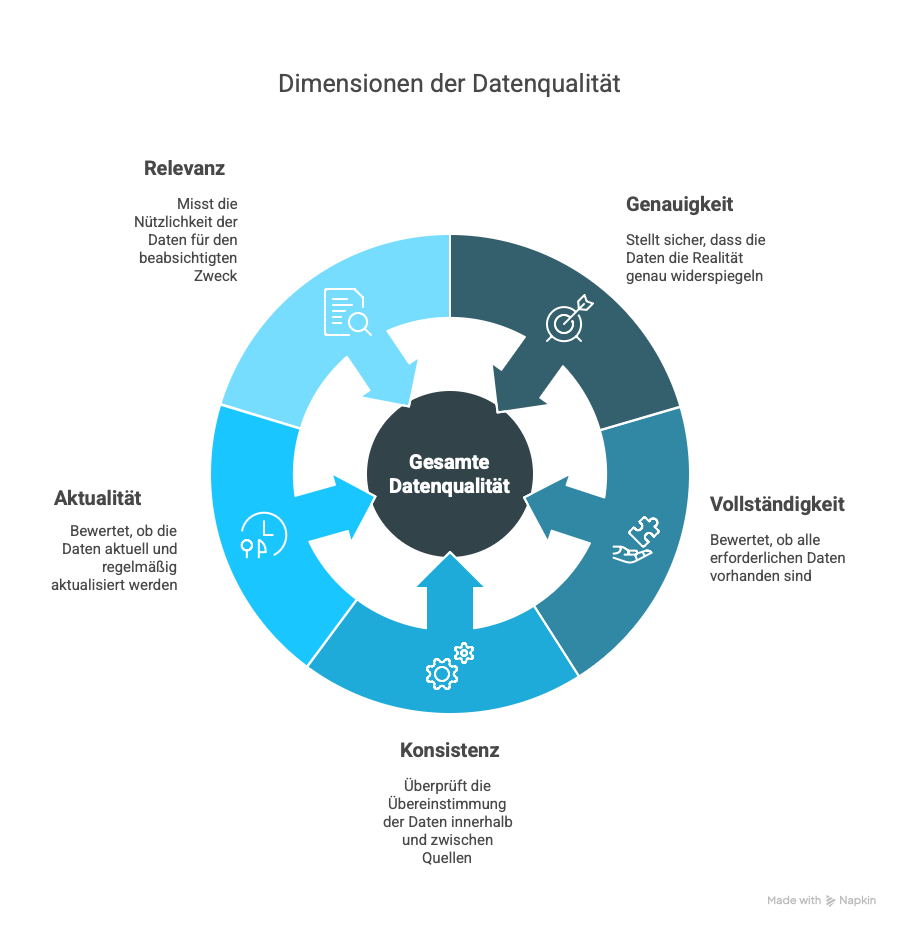
What are the 7 Aspects of Data Quality?
Beyond the basic elements, seven specific aspects can be identified:
- Integrity: structural correctness and consistency of data according to predefined rules
- Uniqueness: freedom from duplicates and redundant information
- Plausibility: credibility of data in view of known facts and patterns
- Accessibility: easy and quick access for authorized users
- Security: protection against unauthorized access, misuse, or loss
- Traceability: ability to trace data back to its source
- Interpretability: clarity and comprehensibility of data for users
Data Quality as the Foundation for Successful AI Implementations
The implementation of AI solutions for market analysis requires a deep understanding of the relationships between data quality and AI performance. Companies must recognize that the quality of their AI-powered analyses directly depends on the quality of the input data.
What are Optimal Data?
Optimal data for AI-powered market analyses are characterized by several key features:
- Representativeness for the target group or relevant market under investigation
- Adequacy in terms of scope and granularity
- Good structure and machine readability
- Temporal relevance according to the analysis requirements
What Types of Data Exist?
In the context of market analysis, various data types can be distinguished:
Structured data follows a predefined schema and is organized in tabular form, such as sales figures or customer information. These data can be relatively easily analyzed and integrated into AI models.
Unstructured data, on the other hand, does not exist in a predefined format. These include texts from social media, customer reviews, or images. They often contain valuable insights but place higher demands on preprocessing.
Time series data represent changes over time and are particularly important for identifying trends and seasonal patterns.
Transaction data document specific events or interactions and often form the backbone of customer analyses and revenue forecasts.
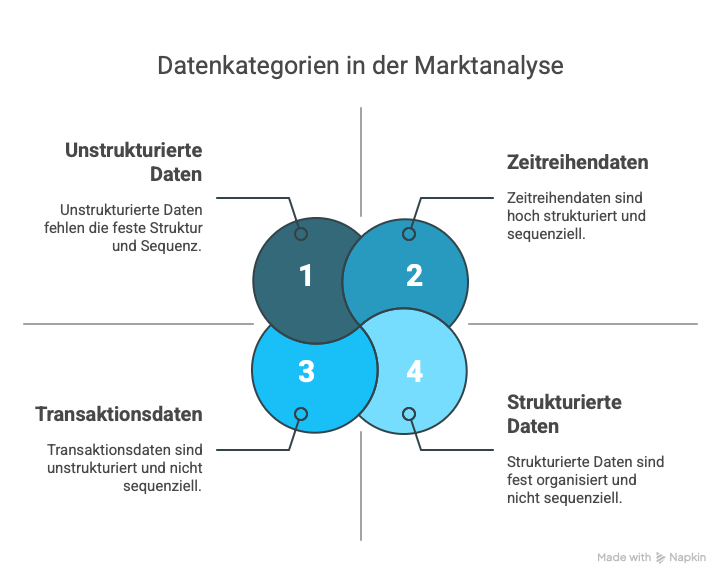
What are the Two Types of Data?
In a more basic categorization, data can be divided into qualitative and quantitative types:
Quantitative data are numerical and measurable, such as sales figures, market shares, or prices. They are particularly well-suited for mathematical analyses and statistical models.
Qualitative data, on the other hand, are descriptive and non-numerical. They include customer feedback, expert opinions, or observations of consumer behavior. These data offer deeper insights into motivations and preferences.
Characteristics and Principles of High-Quality Data
To systematically improve and manage data quality, a clear understanding of the basic characteristics and principles is required.
What are the Characteristics of Good Data Quality?
Good data quality is characterized by several central properties:
Reliability describes the trust that can be placed in the data and their consistency when repeatedly captured under similar conditions. Reliable data lead to reproducible analysis results.
Fitness for purpose refers to the suitability of the data for the specific analysis purpose. High-quality data are tailored to the respective business objectives and analysis requirements.
Precision refers to the level of detail and accuracy of the data. Depending on the use case, different degrees of precision may be required.
Understandability means that the data are clearly documented and easily interpretable. This includes clear definitions and comprehensible metadata.
Accessibility ensures that authorized users can retrieve the needed data in a timely and uncomplicated manner.
What are the 6 Principles of Data Quality?
Six fundamental principles can serve as guidelines for effective data quality management:
- Accuracy: Data must correctly represent the real objects, events, or concepts
- Completeness: All required data elements must be present
- Consistency: Freedom from contradictions within and between datasets
- Timeliness: Importance of timely data
- Relevance: Data must be meaningful for the specific business purpose
- Transparency: Clarity about the origin, processing, and quality of the data
Strategies for Improving Data Quality
Ensuring high data quality requires a systematic approach and continuous efforts.
How Do You Ensure Data Quality?
Ensuring data quality requires a comprehensive approach:
- Implementation of a data quality framework with standards, metrics, and processes
- Regular data audits to verify quality standards
- Data validation at the source for early error detection
- Training and awareness of employees
- Centralized data management with clear governance structures
"The trustworthiness of AI models stands or falls with the quality of the training data. Companies must therefore give top priority to data quality in order to benefit from the advantages of AI-powered market analyses," emphasizes a leading expert in Business Intelligence.
What is the Key to Improving Data Quality?
The key to sustainable improvement of data quality lies in a combination of technological solutions and organizational measures:
Technological solutions such as data profiling tools, quality monitoring systems, and automated cleaning processes can facilitate the identification and resolution of quality problems.
However, a clear data strategy is equally important. It defines the long-term goals of data management, sets priorities, and ensures that data quality initiatives align with corporate objectives.
Why is a data strategy important? It provides a structured approach to managing data resources and ensures that data are treated as a strategic asset. An effective data strategy considers the entire data lifecycle – from collection through storage and processing to analysis and archiving.
A leading consulting firm reports on a remarkable case in which an insurance company was able to increase its cross-selling rate by 35% by optimizing its customer data for AI analyses.
Practical Approaches to Data Optimization for AI Models
The concrete implementation of data quality measures requires practical approaches and tools.
How Do You Optimize Data?
Optimizing data for AI-powered market analyses encompasses several key activities:
- Data cleaning to identify and correct errors
- Data standardization for uniform formats and representations
- Data enrichment through additional relevant information
- Feature engineering to improve AI model performance
- Data reduction for efficient handling of large amounts of data
Upselling and cross-selling strategies particularly benefit from high data quality, as they are based on precise customer understanding.
What Impact Can the Quality of Data Collection Methods Have on the Reliability of Insights?
The quality of data collection methods has a direct influence on the reliability of analysis results:
- Systematic biases can arise from poorly designed collection methods
- The granularity of collection determines the achievable level of detail
- The frequency and regularity influence the detection of temporal patterns
- Contextualization improves the interpretability of the data
Challenges in Data Quality Assurance
Despite the importance of high-quality data, companies face significant challenges in ensuring data quality.
How Do You Fix Poor Data Quality?
Fixing data quality problems requires a structured approach:
- Identification of problem sources through thorough root cause analysis
- Implementation of automated validation rules at the data source
- Conduct data cleaning processes to correct existing problems
- Establishment of continuous monitoring and regular audits
- Training of employees and establishment of clear responsibilities
The implementation of agential AI systems for upselling and cross-selling is fundamentally based on the quality of the underlying data.
What Constitutes Data Quality?
The essence of good data quality can be summarized in several core aspects:
- Fitness for purpose – the suitability of the data for their intended use
- Reliability and trustworthiness for practical use
- Balance between depth of detail and usability
- Consistency across time and systems
- Adaptability to changing requirements
Conclusion: The Path to Reliable AI-Powered Market Analyses
The quality of data is the decisive success factor for AI-powered market analyses. In a time when companies increasingly rely on Artificial Intelligence to achieve competitive advantages, the systematic assurance of data quality becomes a strategic necessity.
The various dimensions of data quality – from accuracy and completeness to timeliness and relevance – form a complex field that requires continuous attention. Companies that invest in data quality create the foundation for trustworthy analyses and informed decisions.
The implementation of a comprehensive data strategy is not a one-time task, but an ongoing process. This process should be based on clear principles, supported by appropriate technologies, and embedded in the organizational culture.
For companies in Germany, traditionally known for their precision and thoroughness, focusing on data quality in AI projects offers a natural extension of these strengths. The careful handling of data not only meets high quality standards but also supports compliance with strict European data protection requirements.
In this sense, investment in data quality is not a cost position but a strategic decision that secures long-term competitive advantages. Companies that translate this insight into concrete measures will be better positioned to exploit the full potential of AI-powered market analyses.
Note: This article is for informational purposes only and does not constitute comprehensive guidance for implementing data quality measures. The optimal approach depends on the specific requirements and framework conditions of your company.
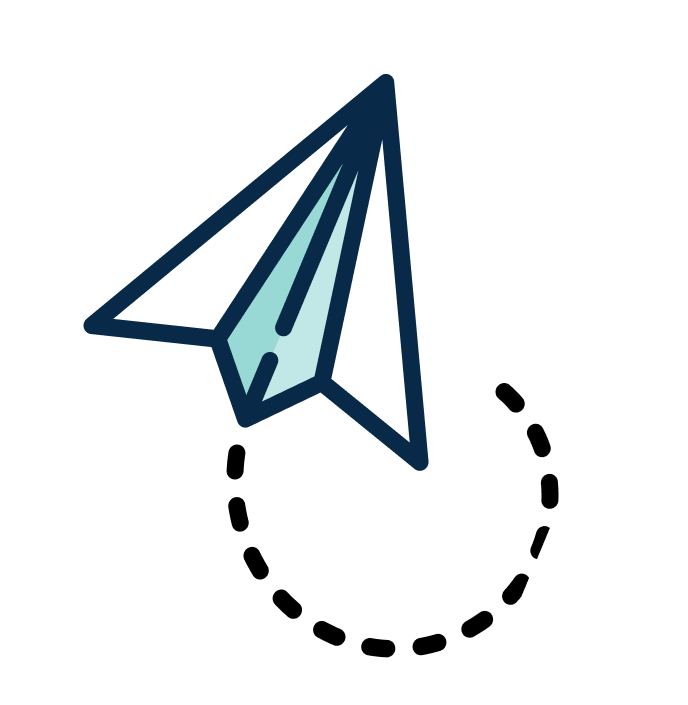