Data-Driven Lead Scoring: How Companies Use AI to Identify Their Best Customers
In today’s data-driven business world, companies face the challenge of identifying the most promising prospects from a vast pool of contacts. This is where data-driven lead scoring comes into play—a systematic approach that leverages artificial intelligence (AI) to help businesses allocate their sales resources more efficiently and significantly improve conversion rates.
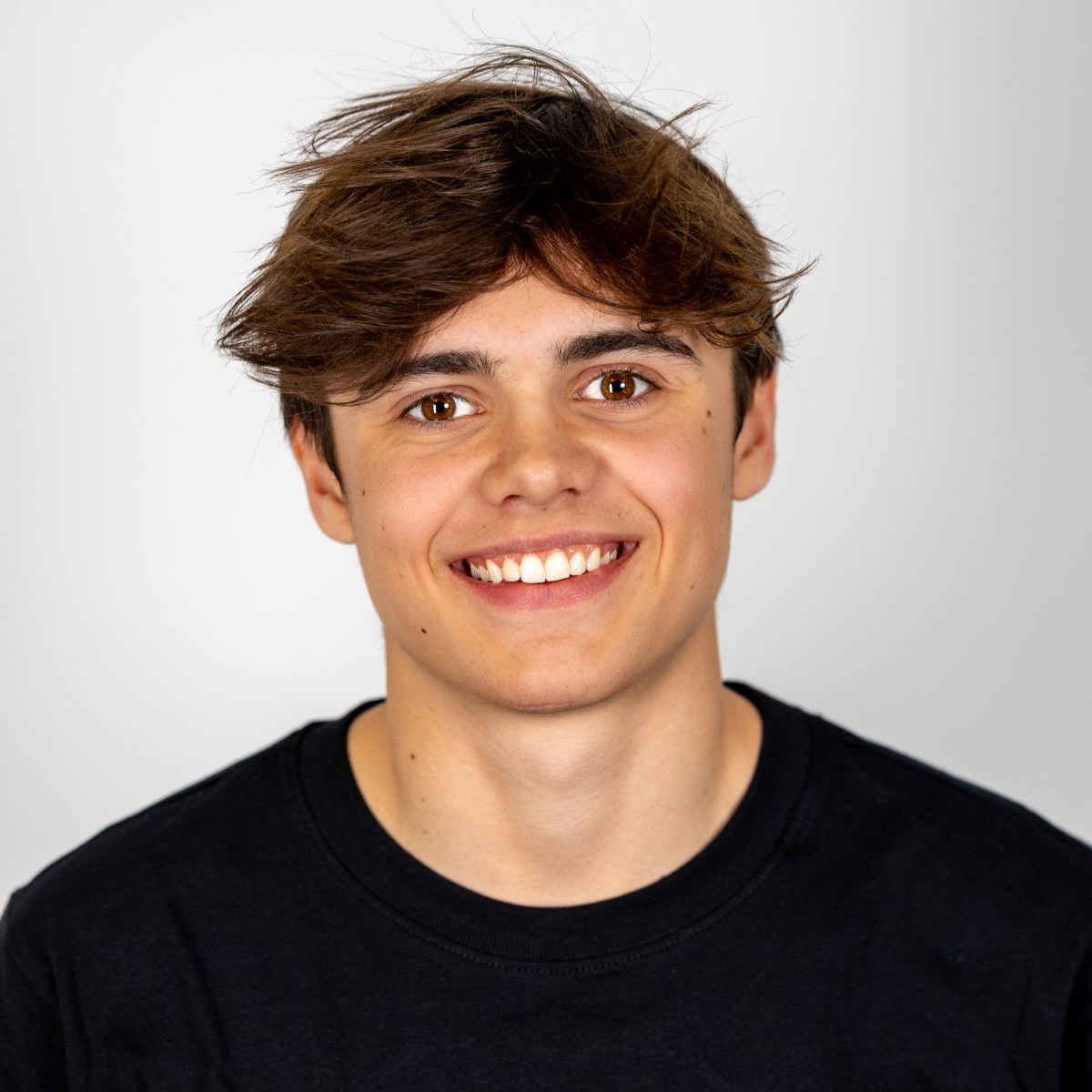
The Basics of Data-Driven Lead Scoring
Lead scoring is more than just a method for evaluating potential customers—it is a strategic tool that can transform the entire sales process.
What Is a Lead Scoring Model?
A lead scoring model is a systematic framework for assessing and prioritizing potential customers based on their likelihood to make a purchase. Traditional models use a point-based system, assigning scores to leads based on certain behaviors or demographic characteristics. Modern data-driven scoring models utilize complex algorithms and machine learning to analyze historical data, identify patterns, and make highly accurate predictions.
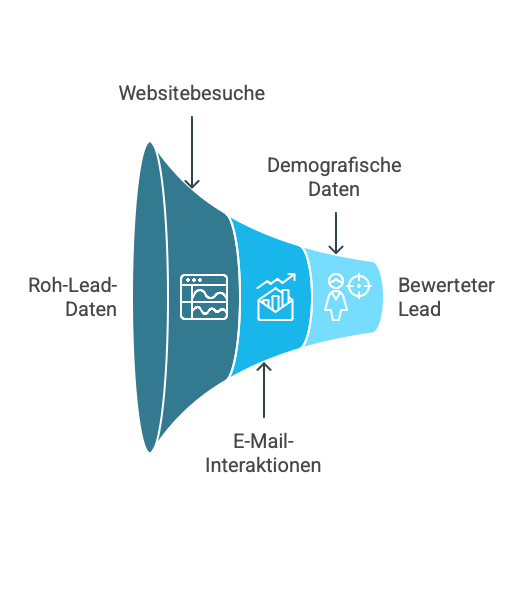
How Is a Lead Score Calculated?
A lead score is calculated by aggregating various data points that signal interest and purchase intent. In a traditional model, points are assigned for explicit factors (such as industry or company size) and implicit factors (such as website visits or whitepaper downloads).
AI-powered models go a step further by dynamically adjusting the weighting of these factors to continuously improve prediction accuracy. They detect patterns and correlations that may not be immediately obvious to humans, thus forecasting conversion likelihood with greater precision.
Categories of Leads
Effectively categorizing leads is crucial for successful lead management. Different types of leads require different approaches in outreach and nurturing.
What Are Qualified Leads?
Qualified leads are potential customers who meet specific criteria that identify them as promising sales candidates. There are different qualification levels:
- Marketing Qualified Leads (MQLs): Have shown enough interest and engagement to be considered potential customers by the marketing team.
- Sales Qualified Leads (SQLs): Have been evaluated by the sales team as ready for direct sales outreach.
- Product Qualified Leads (PQLs): Have already interacted with the product, such as through a free trial.
What Makes a Good Lead?
Good leads are not only qualified but also highly aligned with your ideal customer profile. Characteristics of good leads include high engagement rates, active problem-solving efforts, suitable demographic traits, and clear buying signals such as pricing inquiries or demo requests.
AI-driven lead scoring models can automatically analyze and evaluate these factors to identify the most promising leads.
Implementing Modern Lead Scoring Systems
Implementing an effective lead scoring system requires a strategic approach and integration of various data sources.
How to Develop a Predictive Lead Scoring Model?
A predictive lead scoring model is based on analyzing historical data and identifying patterns that predict successful conversions. Development involves several steps:
- Identify and collect relevant data points.
- Train AI algorithms using this data to recognize patterns.
- Validate and continuously optimize the model.
The advantage of AI-powered models lies in their ability to recognize complex relationships and dynamically adjust the weighting of various factors. This allows them to make predictions that far exceed the capabilities of traditional rule-based systems.

Is a Multidimensional Scoring System Beneficial?
Yes, multidimensional scoring systems offer significant advantages over one-dimensional approaches. They enable a more nuanced evaluation of leads by analyzing and assessing different aspects of customer behavior and profiles separately.
For example, such a system could calculate separate scores for engagement level, alignment with the ideal customer profile, and current purchase intent. This multidimensional view helps sales teams not only determine which leads should be prioritized but also decide which type of outreach would be most effective.
The integration of AI in these multidimensional systems enables even more precise predictions and continuous optimization of scoring models. Predictive functions analyze historical data to identify the key indicators for successful conversions in each dimension.
Why Is Lead Scoring Important?
At a time when businesses are overwhelmed with data and potential customer contacts, lead scoring has become an essential tool for efficient sales processes.
Why Are Leads So Important?
Leads are the lifeblood of any business. They represent potential customers and thus future revenue and growth opportunities. However, not all leads are equally valuable. Some have a higher likelihood of converting into customers, while others may never convert.
In this context, lead scoring becomes a strategic imperative. It enables businesses to allocate resources efficiently by focusing on the most promising leads, leading to higher conversion rates, shorter sales cycles, and ultimately a better ROI for marketing and sales activities.
When Does a Lead Become an Opportunity?
The transition from a lead to an opportunity marks a crucial point in the sales process. This transition typically occurs when a lead meets certain qualification criteria and has demonstrated genuine interest in making a purchase.
Typical indicators of this transition include clearly defined needs, a set budget, a specific timeline for a purchase decision, and identification of decision-makers.
Data-driven lead scoring can predict this transition more precisely by analyzing historical data and recognizing patterns that have led to successful conversions.
Why Is It Important to Prioritize Incoming Leads?
Prioritizing incoming leads is crucial for several reasons:
- Timeliness: The likelihood of a successful interaction drops significantly the longer a lead remains unaddressed.
- Resource Optimization: Sales teams have limited capacity that should be utilized effectively.
- Customer Experience: High-potential leads deserve prompt and personalized engagement.
- Competitive Advantage: In competitive markets, quick responses can make all the difference.
AI-based lead scoring enables automated and precise prioritization in real-time, ensuring that the most valuable leads are always addressed first.
The Future of Lead Scoring with AI
Artificial intelligence is revolutionizing lead scoring, offering entirely new opportunities for businesses to optimize their sales processes.
How Does Leadinfo Work?
Leadinfo systems, particularly AI-based ones, collect and analyze a wide range of data to create comprehensive profiles of potential customers. These systems go beyond traditional tracking methods and use advanced algorithms to detect and interpret behavioral patterns.
Modern Leadinfo platforms can identify website visitors, analyze browsing behavior, integrate social media activities, and track behavioral changes over time to signal purchase readiness.
How Does Lead Scoring Enhance Marketing Automation Effectiveness?
Lead scoring is a core component of modern marketing automation and enhances its effectiveness in several ways:
- Accurate Segmentation: With precise lead scores, marketing teams can segment their audience more effectively and develop personalized campaigns.
- Optimized Lead Nurturing Processes: Lead evaluation enables tailored nurturing campaigns that guide leads towards a purchase decision.
- Efficient Resource Allocation: Marketing budgets can be focused on the most promising segments and channels.
- Seamless Integration with Sales: A well-implemented lead scoring system creates a common language between marketing and sales.
- ROI Measurement and Optimization: By correlating lead scores with actual conversion rates, businesses can better measure the ROI of their marketing activities.
The integration of AI into this process elevates these benefits to a new level. AI-powered systems not only learn from historical data but also make real-time adjustments in response to changing market conditions or customer behaviors.
Conclusion: Leveraging Data for Sales Success
Data-driven lead scoring with AI represents a paradigm shift in how businesses evaluate and prioritize potential customers. By leveraging advanced algorithms, companies can now build predictive models that identify the most promising leads with unparalleled accuracy.
The advantages of this approach are numerous: higher conversion rates, shorter sales cycles, more efficient resource allocation, and increased revenue. Especially in an era of overwhelming data, intelligent lead scoring provides a decisive competitive advantage.
While AI integration in lead scoring is a technological advancement, it is also a strategic necessity. Companies that adopt these technologies early will be better positioned to anticipate market trends and understand customer needs.
However, despite all technological progress, one thing remains clear: Lead scoring does not replace human expertise and relationship-building. Instead, it serves as a powerful tool that empowers sales teams to work more effectively. For businesses looking to stay competitive, investing in data-driven lead scoring with AI is no longer optional but a critical step towards sustainable growth.
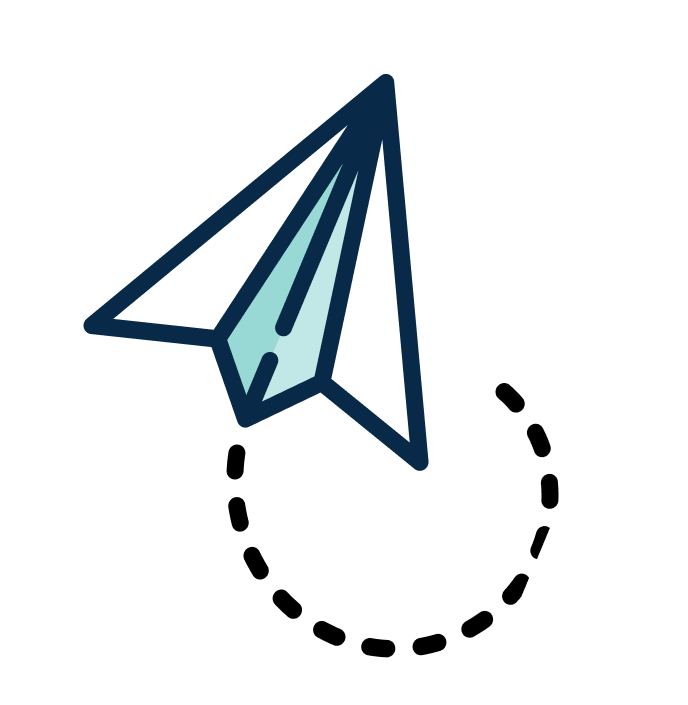