Automated sentiment analysis: What customers really think about your brand
Automated sentiment analysis has evolved from an experimental tool to a strategic business function. It enables companies to understand and respond to the collective voice of their customers - not only based on what they say, but also on what they feel.
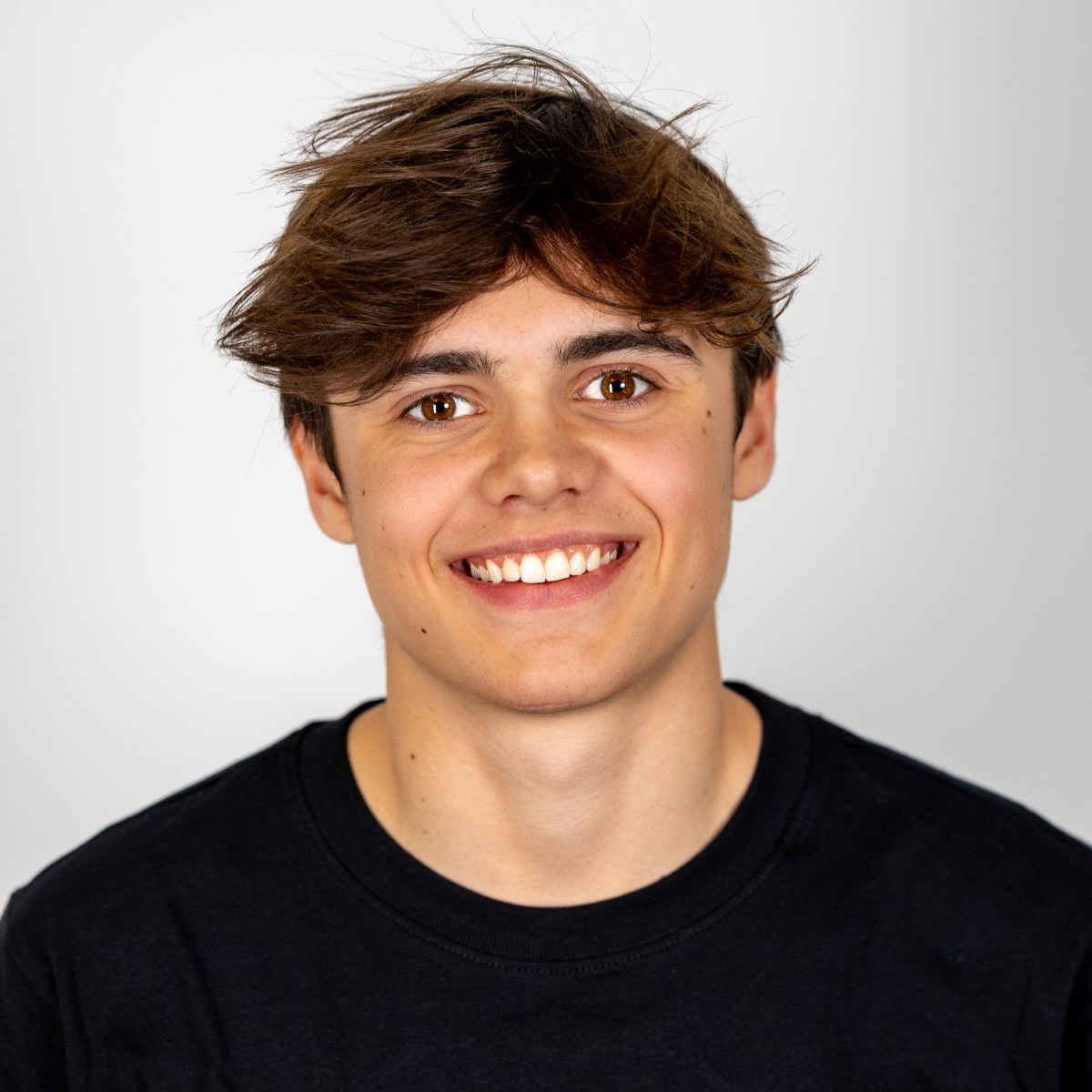
Introduction: Deciphering the hidden voice of your customers
In the digital era, customers leave their mark everywhere - in comment columns, on review platforms, on social media and in support requests. This wealth of customer feedback contains valuable insights that are often lost in the noise of the flood of data. What do customers really think about your brand? Manually evaluating this amount of information is practically impossible - this is where automated sentiment analysis comes into play.
Sentiment analysis, also known as mood analysis, uses artificial intelligence and natural language processing (NLP) to recognize and categorize the tonality and emotional coloring in texts. It transforms unstructured text data into structured insights that help companies take the pulse of their target audience and make data-driven decisions.
What is sentiment analysis, and why is it important?
Sentiment analysis is a technology that uses text analytics to identify and classify the emotional tone and attitude in written content. It goes beyond simply capturing words and captures the mood behind statements - be it positive, negative or neutral. More advanced systems even recognize nuanced emotions such as happiness, frustration, disappointment or enthusiasm.
Understanding customer sentiment is crucial for companies for several reasons. First, it enables a deeper connection with the target audience - beyond sales figures and website traffic. Sentiment analysis uncovers emotional trends that might otherwise go unnoticed.
The technology also allows real-time monitoring of brand perception. Instead of waiting for quarterly surveys, companies can continuously feel the pulse of their online presence and react immediately to critical mood swings.
Last but not least, sentiment analysis creates a competitive advantage through data-supported decision-making. As IBM points out in its analysis on the topic of sentiment analysis to improve customer experience, companies can optimize their products, services and communication strategies in a targeted manner by systematically evaluating customer feedback.
What is the primary purpose of sentiment analysis?
The primary purpose of sentiment analysis is to transform unstructured, qualitative customer data into quantifiable, actionable insights. It serves as a bridge between what customers express and what companies can understand. At its core, it monitors brand perception, identifies problem areas, recognizes trends and measures the impact of marketing campaigns or product launches.
Sentiment analysis not only answers the question “What are customers saying?”, but also “How does it make them feel?”. This emotional dimension is often the key to deeper customer understanding and long-term customer loyalty.
The three main types of sentiment analysis
Sentiment analysis can be divided into different categories, each covering different aspects of sentiment analysis.
Document-based sentiment analysis
Document-based sentiment analysis looks at a text document as a whole and determines the prevailing sentiment throughout the text. This approach is particularly suitable for analyzing product reviews, customer feedback emails or longer social media posts. The result is typically an overall rating that indicates whether the text is predominantly positive, negative or neutral.
The advantage lies in its simplicity and the ability to quickly get an overview of large amounts of customer feedback. The challenge, however, is that nuanced or mixed opinions are not always captured accurately.
Aspect-based sentiment analysis
Aspect-based sentiment analysis breaks down texts into specific aspects or attributes, for each of which a separate sentiment score is then determined. This differentiated approach is particularly valuable for detailed product analyses and the identification of specific potential for improvement.
In practice, this means that in a hotel review, for example, the sentiment can be analyzed separately for aspects such as “room cleaning”, “friendliness of staff” and “value for money”. This gives companies a granular picture of which specific areas are working well and which need to be optimized.
Fine-grained emotional analysis
Fine-grained emotional analysis goes beyond simple polarity (positive/negative/neutral) and identifies specific emotions such as joy, surprise, anger, sadness or fear.
This approach uses advanced machine learning models and linguistic rules to recognize subtle emotional nuances. It is particularly valuable for brands looking to build deeper emotional connections with their customers, as it provides insights into psychological reactions to products or brand communications.
As the Digital Marketing Dictionary points out in its article on brand sentiment analysis, by understanding the specific emotions, companies can refine and personalize their communication strategy.

How does automated sentiment analysis work?
Automated sentiment analysis combines linguistics, statistics and machine learning to decode the emotions behind texts.
Data collection and pre-processing
The first step is to collect relevant text data from various sources such as social media, customer reviews, surveys or support tickets. This raw text data then needs to be pre-processed, which typically involves removing irrelevant information, spelling correction, tokenization and lemmatization.
The quality of the pre-processing has a significant impact on the accuracy of the subsequent analysis. Colloquial language, irony, dialects and multilingual content pose particular challenges.
Rule-based vs. machine learning approaches
Two main methodological approaches are used for the actual sentiment analysis:
Rule-based approaches work with predefined dictionaries and linguistic rules. This involves assigning certain sentiment values to words and phrases. This method is relatively transparent, but reaches its limits with more complex linguistic phenomena such as sarcasm.
Machine learning approaches train algorithms with large quantities of already classified texts. Deep learning models such as BERT, which can process context information much better, are particularly powerful. These approaches require extensive training data, but deliver more precise results, especially for complex linguistic expressions.
In practice, hybrid systems that combine the strengths of both approaches are often used. As Computerwoche explains in its article “What is sentiment analysis?”, these combined approaches enable particularly robust and accurate sentiment analysis.
Differences between sentiment analysis and related concepts
Sentiment analysis is part of a broader spectrum of text analysis techniques. For a comprehensive understanding, it is important to know the differences to related concepts.
Sentiment analysis vs. semantic analysis
Semantic analysis focuses on the meaning of words and sentences and attempts to capture the essence of a statement. It asks: “What is being said?” Sentiment analysis, on the other hand, concentrates on the emotional tonality and asks: “How is it being said?”
In practice, the two forms of analysis often complement each other: semantic analysis helps to identify the topic, while sentiment analysis determines the emotional attitude towards it.
Sentiment analysis vs. emotion analysis
While classic sentiment analysis focuses on polarity (positive, negative, neutral), emotion analysis goes one step further and identifies specific emotions such as joy, surprise, anger, sadness or fear.
Emotion analysis often works with more complex psychological models and finer categorizations. In modern systems, the boundaries are becoming increasingly blurred, as advanced sentiment analysis tools often also offer emotional categorizations.
Difference between opinion and sentiment
An opinion is a subjective belief or view on a particular topic, while a sentiment describes the emotional component or attitude behind that opinion.
Analyzing opinions and sentiments together enables companies to understand not only what views their customers hold, but also the emotional intensity and coloring with which they are held.
The role of AI and machine learning in sentiment analysis
Modern sentiment analysis would be unthinkable without advances in artificial intelligence and machine learning.
How AI improves sentiment analysis
Artificial intelligence, particularly in the form of natural language processing (NLP) and deep learning, has brought several key improvements:
- Better contextual understanding
- Recognition of linguistic subtleties such as irony and sarcasm
- Multimodal analysis of text, images and audio
- Automatic adaptation to changing speech patterns
Which algorithms are used for sentiment analysis?
Various algorithms are used in modern sentiment analysis:
- Naive Bayes classifiers: simple and efficient for basic analysis
- Support Vector Machines (SVM): Powerful for medium-sized data sets
- Recurrent Neural Networks (RNN) and LSTM: Good for longer texts
- Transformer models such as BERT: State-of-the-art for precise sentiment analysis
Is ChatGPT suitable for sentiment analysis?
ChatGPT and similar language models can certainly recognize sentiment in texts and are suitable for:
- Preliminary analysis and proof-of-concepts
- Qualitative evaluations of small amounts of text
- The interpretation of complex linguistic constructs
For systematic, large-scale analyses, however, specialized tools have advantages in terms of precision, quantifiability, integration and visualization. In practice, both approaches can be complementary.
Practical applications of sentiment analysis in the corporate context
Sentiment analysis offers concrete applications with measurable business value.
Brand monitoring and reputation management
Continuous monitoring of brand perception makes it possible to track how the brand is perceived in real time. This is particularly valuable in crisis management: sudden increases in negative mentions can indicate emerging PR crises before they reach critical proportions.
Product development and optimization
By systematically evaluating customer feedback, companies can
- Identify and improve frequently criticized product aspects
- Recognize and further develop particularly valued functions
- Derive new product ideas from customer requests
- Measure reactions to product updates
- Competitor analysis
Sentiment analysis can provide valuable insights into the perception of competitors:
- Identify relative strengths and weaknesses compared to competitors
- Learn from positively evaluated aspects of the competition
- Discover gaps in the market where customers are dissatisfied with existing offerings
Customer service and customer experience
In customer service, sentiment analysis can help to improve the quality of interactions:
- Prioritize customer requests according to urgency
- Support service employees with communication
- Identify recurring problems
- Measure the success of service improvements
The implementation of an automated sentiment analysis solution
The successful implementation of sentiment analysis requires careful planning and execution.
Steps to implementation
- Define goals: First, clarify the business objectives - brand monitoring, product improvement or customer service optimization?
- Identify data sources: Determine relevant sources such as social media, review portals or support tickets. Pay attention to data protection aspects.
- Organize data collection: Develop processes for continuous data collection via APIs, CRM integrations or other methods.
- Make tool selection: Decide between existing solutions or in-house developments based on your specific requirements.
- Configure the system: Customize the system to your needs, train algorithms and define relevant categories.
- Test and optimize: Conduct pilot tests and refine accuracy based on the results.
- Integration into business processes: Link sentiment analysis to existing systems and workflows.
- Continuous improvement: Monitor performance and adapt the system to changing needs.
Tips for successful implementation
- Set realistic expectations: Sentiment analysis is powerful, but not perfect. Expect an accuracy of 70-85% depending on the complexity of the texts analyzed.
- Plan for human review: A human review should be carried out, especially for critical findings.
- Pay attention to data protection: Ensure that your sentiment analysis complies with applicable data protection regulations, especially in the EU.
- Think across departments: The findings of sentiment analysis are valuable for various areas of the company - from marketing to product development and customer service.
Challenges and limitations of sentiment analysis
Despite its power, sentiment analysis also has limitations that companies should be aware of.
Linguistic and cultural challenges
Sentiment analysis reaches its limits with certain linguistic phenomena:
- Irony and sarcasm: statements such as “I just missed that” are often misinterpreted.
- Cultural contexts: Expressions and emotional evaluations vary greatly across cultures.
- Linguistic diversity: Dialects, slang and industry jargon pose particular challenges.
- Multilingualism: Analysis in different languages requires specific models and resources.
Technical limitations
There are also technical limitations:
- Data quality: accuracy depends heavily on the quality of the training data.
- Context understanding: Even advanced algorithms have difficulties with complex contexts.
- Need for updates: Linguistic developments require regular updates of the models.
Ethical considerations
Ethical aspects must also be taken into account when analyzing sentiment:
- Data protection: customer statements must be analyzed in compliance with data protection regulations.
- Transparency: Customers should know when their statements are being analyzed.
- Fairness: Algorithms can reinforce existing prejudices if the training data is distorted.
Conclusion: The future of automated sentiment analysis
Automated sentiment analysis has evolved from an experimental tool to a strategic business function. It enables companies to understand and respond to the collective voice of their customers - not only based on what they say, but also on what they feel. As AI and machine learning continue to evolve, sentiment analysis will become increasingly precise and nuanced. Future systems will be able to bring together not only text, but also images, videos and audio content in a holistic analysis. Integration with other business data will provide deeper insights into the links between customer sentiment and business success.
Companies that invest in sentiment analysis today are not only creating short-term competitive advantage, but also building long-term capabilities to be more customer-centric, more responsive and ultimately more successful. The key question is no longer whether companies should use sentiment analysis, but how they can most effectively integrate this technology into their strategy to identify and meet real customer needs.
Note: This article is for informational purposes only. The implementation of sentiment analysis should take into account the specific requirements of your company and applicable data protection regulations.
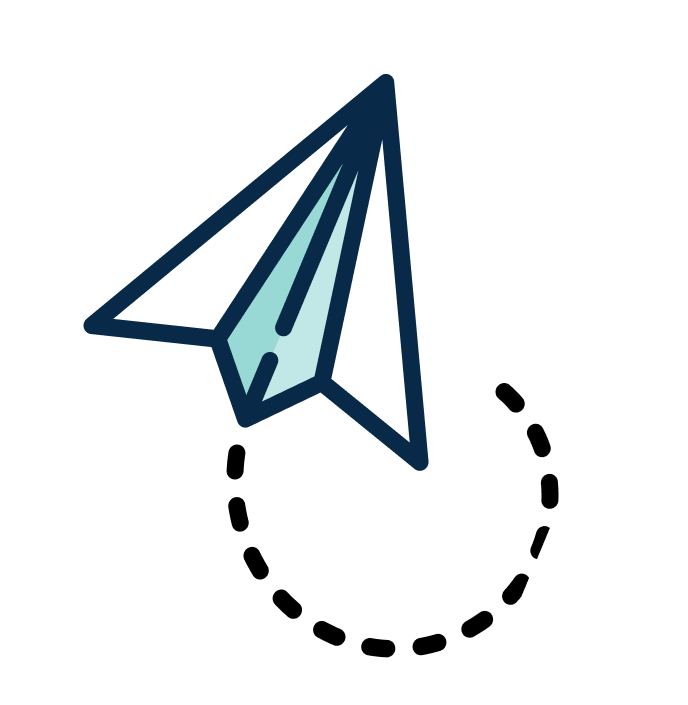